AI/ML Execution Monitoring
Building a scalable monitoring solution for Capital One's ML/AI applications, processes and tools throughout the enterprise.
Engineers need to know what's going on with their models and processes as they run (execute) to ensure they're meeting SLA and business obligations.
Execution monitoring is just that -- it helps modelers react when things go wrong and understand their systems better, in order to troubleshoot and make proactive improvements.
This type of monitoring required the building of an in-house solution that could scale to other ML applications, such as model scoring and system status alerting.
Impact
- Defined scope from business needs, led user research, and developed UX/UI design for an internal AI/ML monitoring tool. Collaborated with product partners to deliver pilot design to 300+ model developers
- Strategically defined experience of multiple features, like AI anomaly detection and automated notifications, for future releases
Role
Team
Time
skills
DISCOVER
Objective
Research and develop a UX/UI solution that allows users to view all services and jobs running on Capital Ones machine learning platform, information about their status, the creation of dashboard views and alerts at different scopes.
Contribution
Translated business needs into project brief, build moderator/user guide, recruited model developers and documented existing constraints.
Result
Aligned on project scope with partners, kicked off research and established design objectives
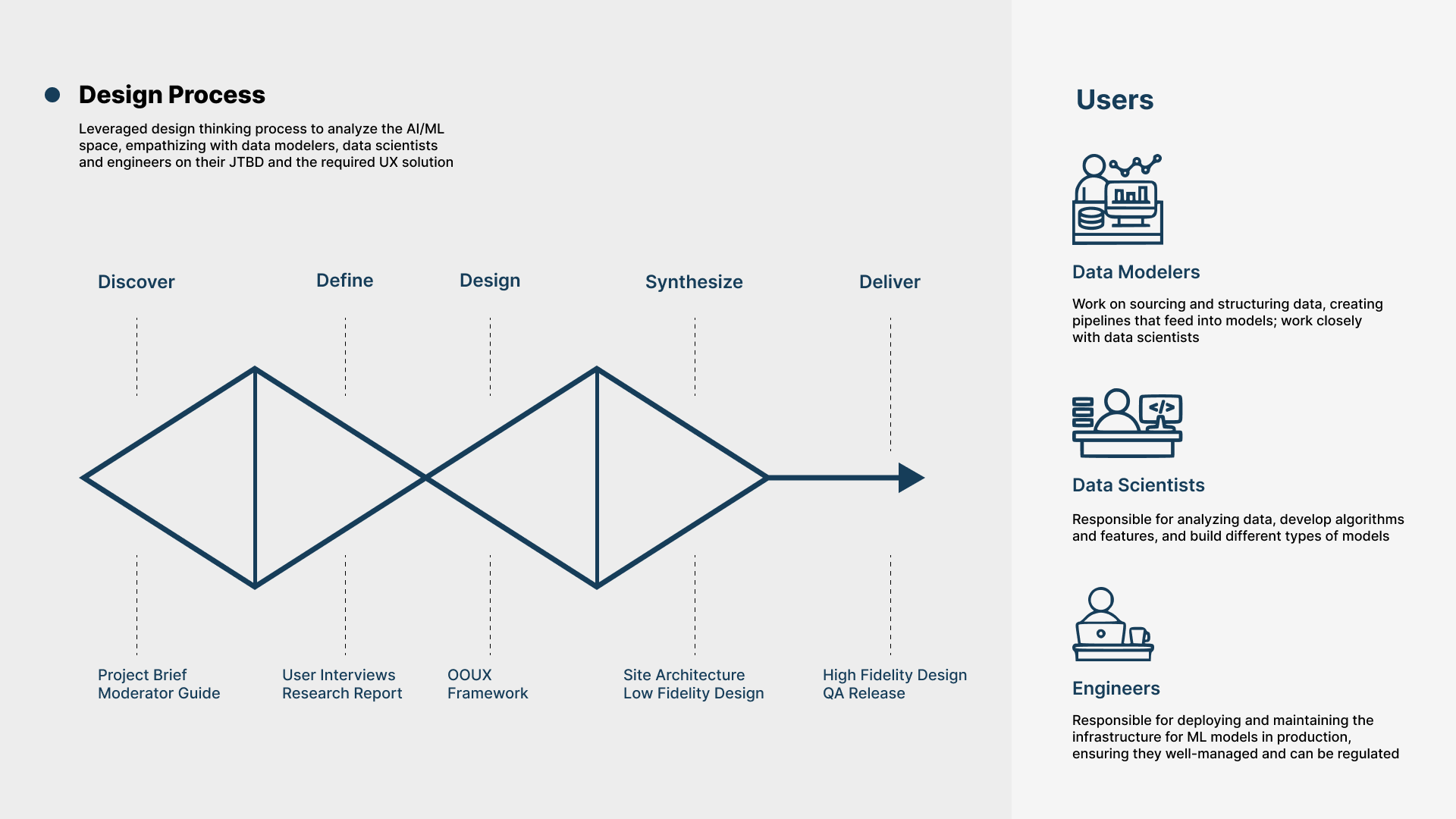

Define
Objective
Synthesize collected interviews into prioritized set of recommendations and prototype
Contribution
Moderated and led 10 interviews, distilled information into research report, documented findings and provided strategic recommendations
Result
Research report was leveraged in prioritization conversations with partners

Design
Objective
Create a scalable design and back-end system that allows for incremental roll out of features.
Contribution
Built an object-oriented UX (OOUX) framework and approach to collaborate with product and tech partners. Delivered multiple data requirements and hierarchy of components.
Result
Prioritized a set of features, like anomaly detection, email notifications, rules engine, etc. and the technical data requirements needed to support those features.
.png)
.png)

Synthesize
Objective
Test the OOUX framework as a low fidelity design, gather feedback from users on the data elements and information architecture
Contribution
Led 5 workshop sessions with model developers to gather feedback on early prototype design, synthesized findings into refined visual
Result
Developed clear understanding of UX/UI design and necessary data elements.
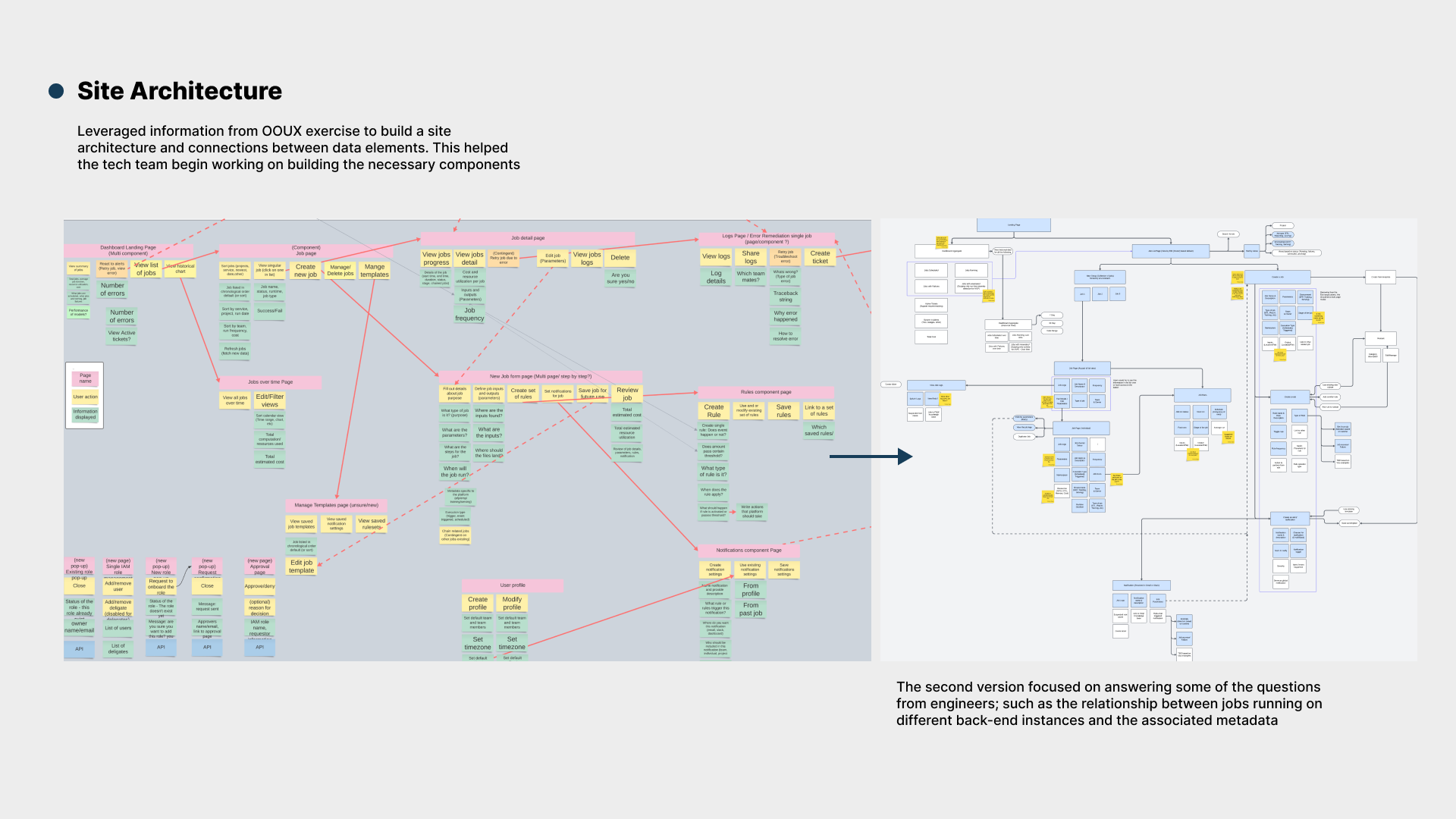
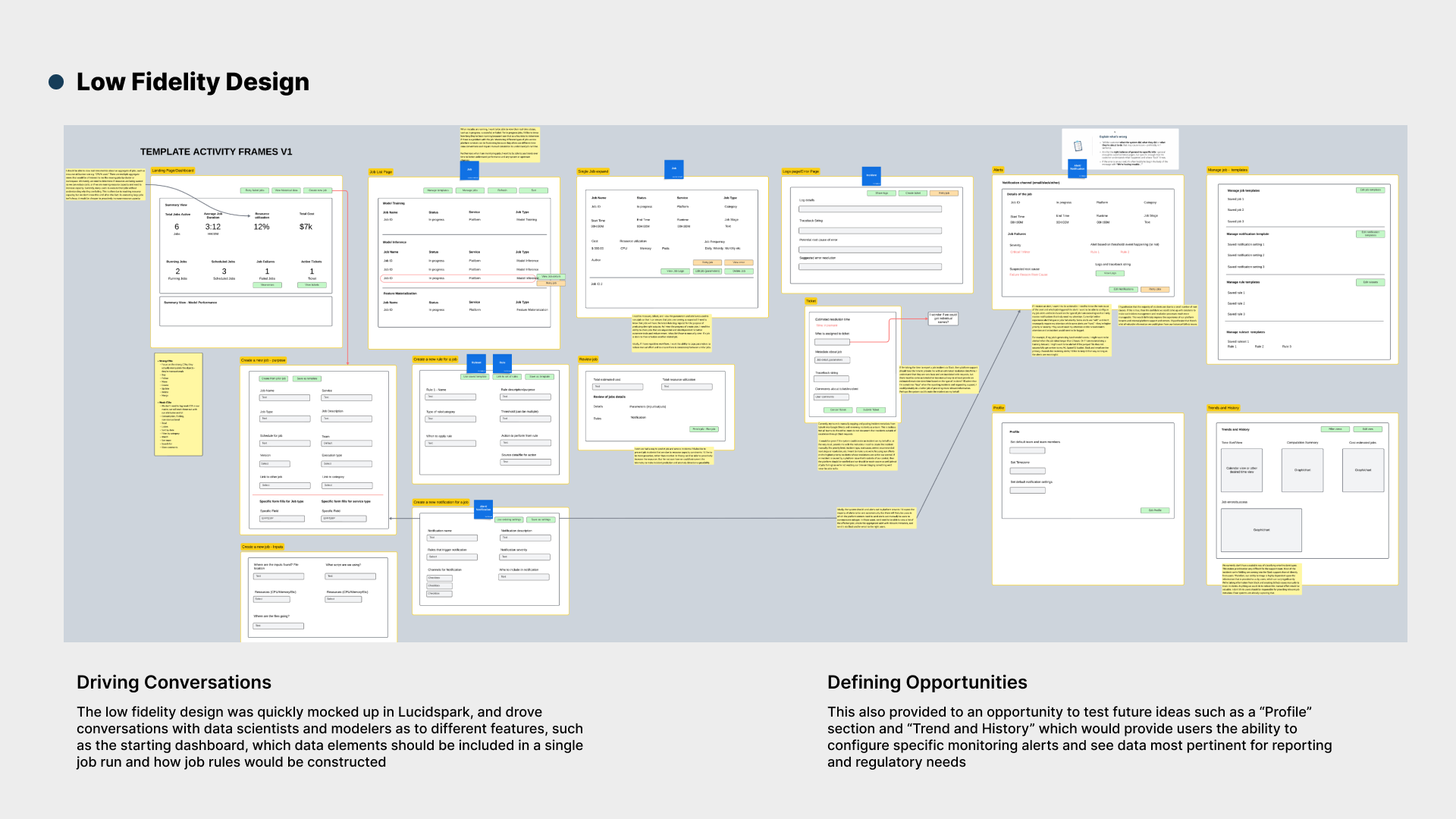
Deliver
Refine the design based on feedback and design expertise into QA pilot
- Built a high fidelity, click through prototype of the experience in Figma.
Created a hand off document. Worked with product and tech to prioritize features based on data feasibility. - Limited release of central dashboard for execution monitoring compute jobs for 300+ model developers

.png)
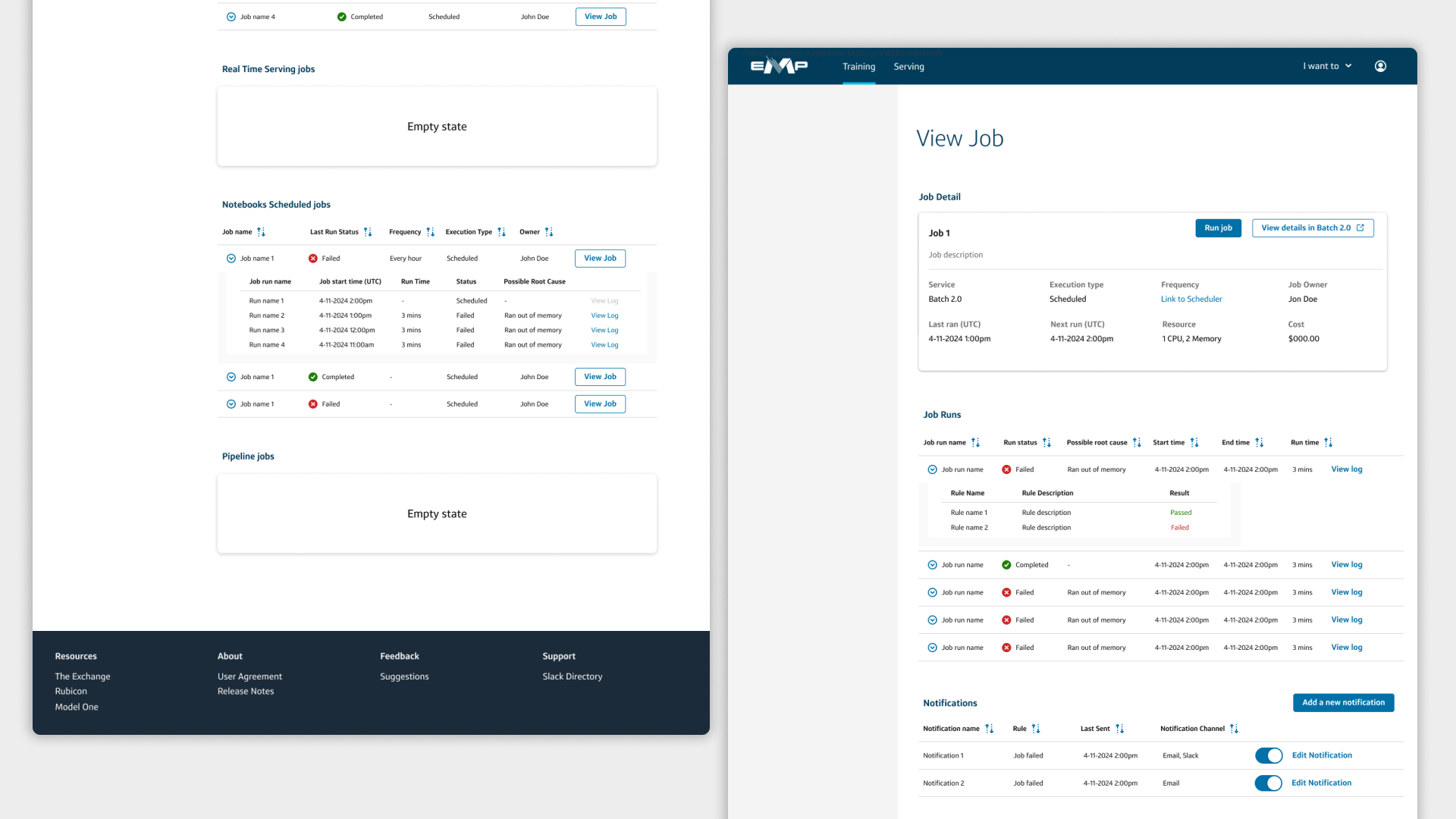